Naked Data
It is hard to explain why data management is still an unruly discipline. Two articles I read recently which on first reading appeared to be unrelated, actually have a connection and help shed some light on the unruliness. The connection is that we work around the shortcomings of the current technologies. This lead me to an idea what a solution would look like, if we are to better support the way humans work with information. The current stack of technologies feel crude, it resembles assembling a wedding cake with an excavator.
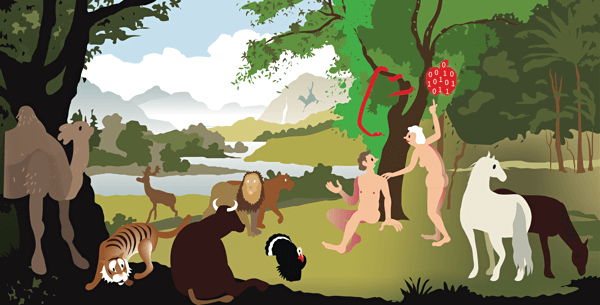
First I read Barry Devlin’s article on context, where he introduced the term ‘naked data’. The article emphasises the importance of context of information and how we struggle with this in technology. Then I read the two part article (part 1 and part 2) from Noreen Kendle where she analyses the sorry state of data management. Of the fundamental factors she describes, the “business – data connection” surfaces again and again. In short, the “business – data connection” is the familiarity people have with information and, as a result, the amount of usefulness they feel for that information in a business environment.
The fundamental challenges of using information
After decades of computerised decision support, you would assume we understand what it takes to manage data. In reality, we look away when someone utters the dreaded words “data governance”. In Noreen Kendle’s words, the chasm in the “business – data connection” keeps growing.
There is an explanation for this, which the diagram below helps explain.
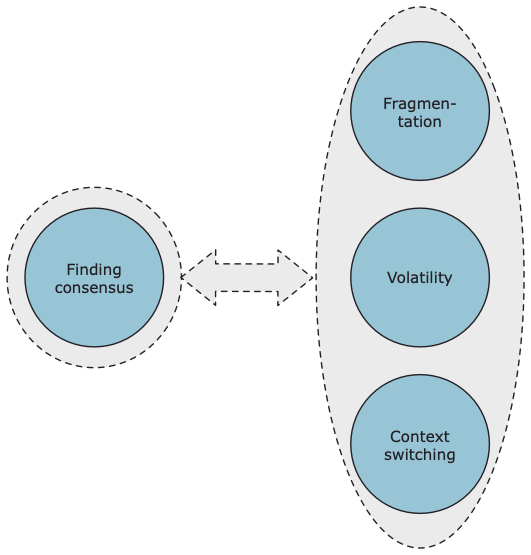
Both fragmentation and volatility have increased exponentially as the ability to store and process huge amounts of data increases. Fragmentation is the scattering of information across an ever increasing amount of data stores. Fragmentation also reflects the number of people with different skills involved in the chain of information provisioning. Volatility is the amount of change in both information demand and in the technological data landscape.
Over time, we have gained the ability to connect to more data repositories. At the same time, technology has made new categories of data collection possible, for instance data from sensors in a smartwatch. While having all this new data may have been expected to lead to more careful curation of that data, actually the opposite has happened and we are simply overwhelmed.
In the 70s, whole staff departments in companies where dedicated to gathering and analysing data, on paper. That was a laborious job and data was expensive. As a staffer, you needed both to have an intimate knowledge of the business challenges and to know where to look for data to get answers. Technological progression has led to hyper specialisation. The journey from data origin to insight and use, now involves multiple people at various stages in the process, each one of whom has different and very specialised skills. The single staffer has been replaced with three or four different people. As one of those people, you feel responsible for the part that you are doing, but you have no idea to what end result your work has contributed. Every day, I meet developers who have no clue to the urgency of the business challenges they work on. Similarly, the business user applying the information often has no clue what that data journey looks like. It is ‘those people from IT’ that do ‘incomprehensible stuff that takes forever’.
Fragmentation and volatility are the result of the technological progress and the effects progress has had on the changes in structure of businesses and markets. What has been lacking is the evolution to a fitting governance model to address this chasm in the ‘business – data connection’. We have been trying to address the chasm with more technology, and new developments like AI assisted data mapping are definitely helping us make more sense of the staggering amounts of data items captured. However, the problem is rooted in human collaboration. Connected architecture is a model which acknowledges this and tries to point to a data management approach that fits today’s world of omnipresent data.
What is taken for granted is the inherent fundamental challenge of dealing with data, which is the ability to use and reuse data in different contexts. This is where technology fails miserably in supporting us.
Context is everything
Data is the result of information recorded within a certain context. Temperature measured is data, but the context can be medical, meteorological or something as simple as barbecuing a steak. If we want to analyze thousands of steaks baked on a wide array of BBQ equipment to determine which BBQ delivers the most stable results, temperature is one of the variables we can use. The context of comparing BBQ equipment is different to the use case of knowing when a steak has been grilled to perfection. This is context switching and we do it all the time, often unnoticed and without any problems. The trouble starts when a group of us has to reach consensus on what is actually the best BBQ.
So how does context relate to data captured? Take for example the measurement of heart rate in beats per minute. Under what conditions was the heart rate captured? Is someone in a deep sleep or active? If active, what was the source? Hospital equipment, for monitoring the medical rehabilitation of a patient after heart surgery or a Fitbit? If it was from a Fitbit, was it captured when the person was riding their bike to work on a sunny Spring morning? , Or on a windy fall day, with head wind, lots of grocery shopping in their bags, and extra stress from knowing they’re running late to pick up the kids from school?
The naked data is the measure of heart rate measure in BPM at a specific point in time, but all the other stuff is context. And context is everything when selecting data or when analysing data. Use of data will add new context. Are you studying the resistance of new bike tires for urban bikes and using heart rate measures as a variable to measure the amount of strain on the biker? Or are you investigating unexpected heart failure in young adults under stress? That would certainly make a difference to how you treat your data.
Context is everything. And the databases we use to capture and crunch data are notoriously bad at capturing context or separating the context from the naked data. In the complexity of information projects series, I dive into what makes gathering analytical information such a tedious process. From a data management point of view, in terms of context, the technology we have at our disposal is mediocre compared to what our human brains can process.
How could technology benefit us instead of hampering us?
Naked data without context is useless. If we want to reinterpret data for any reason, we are forced to endlessly copy and transform data across data stores or within our databases. To make matters worse, the context we can capture and store in a database, together with the data, is limited. You need to be a very experienced IT minded person with a lot of empathy for present and future business challenges to design and operate the machinery needed to analyse data.
So what would the solution have to look like, if it were going to close the gap of the ‘business – data connection’?
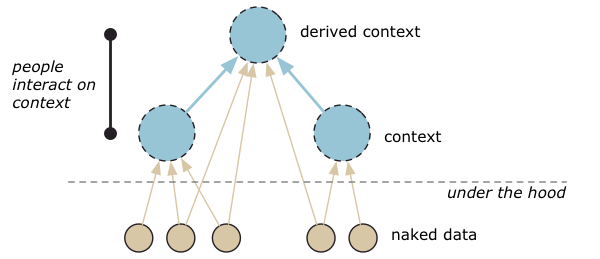
The technology would enable us to only have to interact with contextualized information. The connection of naked data to the context would all be handled under the hood without human intervention. Context is a wide array of types of information. The technology must be able to capture it all and let us interact with it:
- Information origin – the method of capturing information, where it was captured, when it was captured, why it was captured and the amount of variation in the capture.
- Information meaning – the description of what the naked data represents.
- Information purpose – the conditions the information was captured under or transformed to. To what purpose was the information captured or transformed? Crucial information if we want to select and filter out data.
- Information consensus – capturing the different opinions of people on the meaning of information.
Does this sound like a far-fetched idea? I don’t think it is. Your average Word document or e-mail system can capture all of the above mentioned information, but your average word document or e-mail system doesn’t separate the context from the naked data, making it almost impossible to filter and aggregate information to another, new, context. Capturing the new, resulting context is certainly not an option.
Given the technological progress we are currently making, you would expect that analytical systems are also progressing, become fit for purpose so they can mirror the way humans interact with information. I’m afraid that is still a distant point on the horizon.